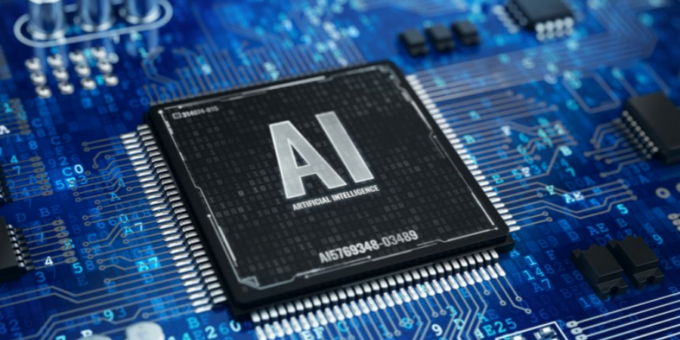
In recent years, artificial intelligence (AI) has advanced in astonishing ways, transforming industries from healthcare to finance. But as algorithms get smarter and data sets grow larger, the hardware that supports AI must also evolve. Enter a new wave of AI hardware innovations—powerful processors, accelerators, and specialized chips designed to handle the massive computational needs of machine learning (ML). Here’s how these breakthroughs in AI hardware are fueling the next generation of intelligent machines and unlocking new possibilities in machine learning.
1. AI-Specific Processors: Tailored for Intense Computation
Traditional CPUs can struggle with the demands of deep learning and neural networks. New processors designed specifically for AI workloads, such as Google’s TPU (Tensor Processing Unit) and NVIDIA’s A100 GPUs, handle these complex tasks more efficiently.
- Why It Matters: AI-specific processors optimize performance and reduce energy consumption, crucial for large-scale ML projects.
- Real-World Example: NVIDIA’s A100 GPU is used in data centers worldwide, enabling faster AI training and inference tasks that power services like real-time speech recognition and image analysis.
Takeaway: Purpose-built AI processors deliver the speed and power needed for cutting-edge ML applications.
2. Neuromorphic Computing: Mimicking the Human Brain
Neuromorphic chips, inspired by the architecture of the human brain, use interconnected neurons to process information more efficiently. These chips aim to make AI models faster and more energy-efficient by handling computations similarly to the human brain.
- Why It Matters: Neuromorphic computing enables low-power, real-time processing, ideal for mobile and edge AI applications.
- Real-World Example: Intel’s Loihi chip uses neuromorphic design principles to create low-energy, high-performance processing solutions that can run advanced AI algorithms on compact devices.
Takeaway: Neuromorphic computing could make AI more efficient and accessible for a range of consumer electronics.
3. Quantum Computing: A Leap Forward in Processing Power
Quantum computers promise to perform calculations far beyond the reach of classical computers, particularly in the realm of machine learning. By using qubits and the principles of quantum mechanics, quantum computing offers unprecedented processing power.
- Why It Matters: Quantum computing could accelerate AI tasks like data processing, encryption, and pattern recognition, making ML models faster and more accurate.
- Real-World Example: Google and IBM are pushing quantum computing’s limits, aiming to create systems that can solve ML problems beyond the reach of today’s supercomputers.
Takeaway: Quantum computing is poised to tackle AI challenges that are currently unsolvable, opening doors to transformative breakthroughs.
4. Edge AI Hardware: Bringing AI Processing Closer to the Source
Edge AI hardware enables real-time data processing on devices like smartphones, cameras, and drones, reducing the need to send data to cloud servers. This local processing approach is ideal for applications where low latency is critical.
- Why It Matters: By processing data on-site, edge AI hardware supports faster responses, lower bandwidth needs, and better privacy.
- Real-World Example: Apple’s Neural Engine in its latest devices allows for real-time face recognition and AR processing without needing cloud support, ensuring faster and more secure processing.
Takeaway: Edge AI is crucial for applications requiring immediate responses, like autonomous vehicles and smart city solutions.
5. Memory Innovations: Speeding Up Data Access
AI and ML require vast amounts of data, making memory access speeds a critical factor in processing efficiency. Innovations like High Bandwidth Memory (HBM) and 3D-stacked DRAM are allowing AI systems to access data faster than ever.
- Why It Matters: Faster memory reduces bottlenecks in AI workloads, helping ML models to train and infer more rapidly.
- Real-World Example: Samsung’s HBM technology is used in data-intensive applications like image processing, allowing quicker and more efficient data transfer in AI systems.
Takeaway: Memory innovations support AI’s ever-increasing data needs, making systems faster and more effective.
6. Application-Specific Integrated Circuits (ASICs): Custom Hardware for AI Tasks
ASICs are custom-designed for specific tasks, such as machine learning inference. Unlike general-purpose CPUs or GPUs, ASICs are specialized for particular functions, making them more efficient for high-frequency AI workloads.
- Why It Matters: ASICs reduce energy use and increase processing speed, making them ideal for large-scale, dedicated AI applications.
- Real-World Example: Google’s TPUs are a form of ASIC, designed specifically for deep learning applications and heavily used in Google’s AI services.
Takeaway: ASICs provide a highly efficient solution for dedicated AI tasks, particularly in data centers.
7. FPGA Advances: Flexible and Efficient AI Hardware
Field-Programmable Gate Arrays (FPGAs) are flexible chips that can be reprogrammed as needed, making them adaptable to evolving AI requirements. While not as powerful as ASICs, FPGAs offer a balance of flexibility and efficiency.
- Why It Matters: FPGAs allow businesses to adapt hardware to new AI models without needing new hardware each time.
- Real-World Example: Microsoft Azure uses FPGAs to optimize AI processing across its cloud services, providing scalable, flexible solutions for its clients.
Takeaway: FPGAs are ideal for applications that require adaptability, particularly in dynamic fields like AI research.
8. AI-Enhanced Hardware Security
AI is also improving hardware security by detecting and defending against potential cyber threats. AI-driven security solutions can spot anomalies and attacks that traditional methods might miss.
- Why It Matters: As AI systems manage sensitive data, secure hardware ensures data protection and compliance.
- Real-World Example: IBM’s AI-powered hardware security systems monitor for unusual activity, providing an extra layer of defense in sensitive applications like finance.
Takeaway: AI-enhanced security solutions are essential for protecting sensitive AI-driven systems and data.
9. Energy-Efficient Chips: Reducing the Environmental Impact of AI
AI training is computationally intensive and often energy-draining. Energy-efficient chips, designed to reduce the power consumption of AI models, are becoming increasingly important as the demand for AI grows.
- Why It Matters: With more companies using AI, reducing energy use is both environmentally responsible and cost-effective.
- Real-World Example: NVIDIA’s Jetson chips, designed for edge AI applications, consume minimal power, making them ideal for portable, battery-powered devices.
Takeaway: Energy-efficient chips align AI advancement with sustainability goals, making technology greener.
10. Optical AI Processing: A Glimpse into the Future
Optical AI processing, using light instead of electricity for computation, is an emerging area that holds immense potential. This technology could make AI processing faster and more energy-efficient.
- Why It Matters: Optical processing could handle complex AI computations at unprecedented speeds with less energy use.
- Real-World Example: Researchers are developing optical processors that could revolutionize data centers, offering a glimpse of what AI hardware could look like in the future.
Takeaway: Optical AI processing could redefine AI hardware, offering incredible speed and efficiency for high-performance tasks.
Conclusion
As we enter 2024, AI hardware innovation is advancing faster than ever, with specialized processors, quantum computing, neuromorphic chips, and more. Each of these technologies plays a unique role in enabling smarter, faster, and more sustainable AI applications. For businesses, developers, and consumers alike, understanding these hardware trends is essential to staying ahead in an increasingly AI-driven world. Embracing these cutting-edge solutions not only unlocks new capabilities but also positions organizations to lead in the AI revolution.